 |
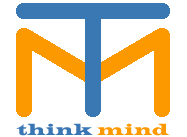 |
InfoSys 2025 Congress March 09, 2025 to March 13, 2025 - Lisbon, Portugal
- ICNS 2025, The Twenty-Second International Conference on Networking and Services
- ICAS 2025, The Twenty-Second International Conference on Autonomic and Autonomous Systems
- ENERGY 2025, The Fifteenth International Conference on Smart Grids, Green Communications and IT Energy-aware Technologies
- WEB 2025, The Thirteenth International Conference on Building and Exploring Web Based Environments
- DBKDA 2025, The Seventeenth International Conference on Advances in Databases, Knowledge, and Data Applications
- SIGNAL 2025, The Tenth International Conference on Advances in Signal, Image and Video Processing
- BIOTECHNO 2025, The Seventeenth International Conference on Bioinformatics, Biocomputational Systems and Biotechnologies
- AIHealth 2025, The Second International Conference on AI-Health
InfoWare 2025 Congress March 09, 2025 to March 13, 2025 - Lisbon, Portugal
- ICCGI 2025, The Twentieth International Multi-Conference on Computing in the Global Information Technology
- ICWMC 2025, The Twenty-Second International Conference on Wireless and Mobile Communications
- VEHICULAR 2025, The Fourteenth International Conference on Advances in Vehicular Systems, Technologies and Applications
- INTERNET 2025, The Seventeenth International Conference on Evolving Internet
- COLLA 2025, The Fifteenth International Conference on Advanced Collaborative Networks, Systems and Applications
- INTELLI 2025, The Fourteenth International Conference on Intelligent Systems and Applications
- VISUAL 2025, The Tenth International Conference on Applications and Systems of Visual Paradigms
- HUSO 2025, The Eleventh International Conference on Human and Social Analytics
- BRAININFO 2025, The Tenth International Conference on Neuroscience and Cognitive Brain Information
DataSys 2025 Congress April 06, 2025 to April 10, 2025 - Valencia, Spain
- AICT 2025, The Twenty-Second Advanced International Conference on Telecommunications
- ICIW 2025, The Twentieth International Conference on Internet and Web Applications and Services
- ICIMP 2025, The Twentieth International Conference on Internet Monitoring and Protection
- SMART 2025, The Fourteenth International Conference on Smart Cities, Systems, Devices and Technologies
- IMMM 2025, The Fifteenth International Conference on Advances in Information Mining and Management
- INFOCOMP 2025, The Fifteenth International Conference on Advanced Communications and Computation
- MOBILITY 2025, The Fifteenth International Conference on Mobile Services, Resources, and Users
- SPWID 2025, The Eleventh International Conference on Smart Portable, Wearable, Implantable and Disability-oriented Devices and Systems
- ACCSE 2025, The Tenth International Conference on Advances in Computation, Communications and Services
ComputationWorld 2025 Congress April 06, 2025 to April 10, 2025 - Valencia, Spain
- SERVICE COMPUTATION 2025, The Seventeenth International Conference on Advanced Service Computing
- CLOUD COMPUTING 2025, The Sixteenth International Conference on Cloud Computing, GRIDs, and Virtualization
- FUTURE COMPUTING 2025, The Seventeenth International Conference on Future Computational Technologies and Applications
- COGNITIVE 2025, The Seventeenth International Conference on Advanced Cognitive Technologies and Applications
- ADAPTIVE 2025, The Seventeenth International Conference on Adaptive and Self-Adaptive Systems and Applications
- CONTENT 2025, The Seventeenth International Conference on Creative Content Technologies
- PATTERNS 2025, The Seventeenth International Conference on Pervasive Patterns and Applications
- COMPUTATION TOOLS 2025, The Sixteenth International Conference on Computational Logics, Algebras, Programming, Tools, and Benchmarking
- BUSTECH 2025, The Fifteenth International Conference on Business Intelligence and Technology
- AIVR 2025, The Second International Conference on Artificial Intelligence and Immersive Virtual Reality
NexComm 2025 Congress May 18, 2025 to May 22, 2025 - Nice, France
- ICDT 2025, The Twentieth International Conference on Digital Telecommunications
- SPACOMM 2025, The Seventeenth International Conference on Advances in Satellite and Space Communications
- ICN 2025, The Twenty-Fourth International Conference on Networks
- ICONS 2025, The Twentieth International Conference on Systems
- MMEDIA 2025, The Seventeenth International Conference on Advances in Multimedia
- PESARO 2025, The Fifteenth International Conference on Performance, Safety and Robustness in Complex Systems and Applications
- CTRQ 2025, The Eighteenth International Conference on Communication Theory, Reliability, and Quality of Service
- ALLDATA 2025, The Eleventh International Conference on Big Data, Small Data, Linked Data and Open Data
- SOFTENG 2025, The Eleventh International Conference on Advances and Trends in Software Engineering
DigitalWorld 2025 Congress May 18, 2025 to May 22, 2025 - Nice, France
- ICDS 2025, The Nineteenth International Conference on Digital Society
- ACHI 2025, The Eighteenth International Conference on Advances in Computer-Human Interactions
- GEOProcessing 2025, The Seventeenth International Conference on Advanced Geographic Information Systems, Applications, and Services
- eTELEMED 2025, The Seventeenth International Conference on eHealth, Telemedicine, and Social Medicine
- eLmL 2025, The Seventeenth International Conference on Mobile, Hybrid, and On-line Learning
- eKNOW 2025, The Seventeenth International Conference on Information, Process, and Knowledge Management
- ALLSENSORS 2025, The Tenth International Conference on Advances in Sensors, Actuators, Metering and Sensing
- SMART ACCESSIBILITY 2025, The Tenth International Conference on Universal Accessibility in the Internet of Things and Smart Environments
IARIA Congress 2025, The 2025 IARIA Annual Congress on Frontiers in Science, Technology, Services, and Applications July 06, 2025 to July 10, 2025 - Athens, Greece
DigiTech 2025 Congress July 06, 2025 to July 10, 2025 - Athens, Greece
- DIGITAL 2025, Advances on Societal Digital Transformation
- IoTAI 2025, The Second International Conference on IoT-AI
- GPTMB 2025, The Second International Conference on Generative Pre-trained Transformer Models and Beyond
NexTech 2025 Congress September 28, 2025 to October 02, 2025 - Lisbon, Portugal
- UBICOMM 2025, The Nineteenth International Conference on Mobile Ubiquitous Computing, Systems, Services and Technologies
- ADVCOMP 2025, The Nineteenth International Conference on Advanced Engineering Computing and Applications in Sciences
- SEMAPRO 2025, The Nineteenth International Conference on Advances in Semantic Processing
- AMBIENT 2025, The Fifteenth International Conference on Ambient Computing, Applications, Services and Technologies
- EMERGING 2025, The Seventeenth International Conference on Emerging Networks and Systems Intelligence
- DATA ANALYTICS 2025, The Fourteenth International Conference on Data Analytics
- GLOBAL HEALTH 2025, The Fourteenth International Conference on Global Health Challenges
- CYBER 2025, The Tenth International Conference on Cyber-Technologies and Cyber-Systems
SoftNet 2025 Congress September 28, 2025 to October 02, 2025 - Lisbon, Portugal
- ICSEA 2025, The Twentieth International Conference on Software Engineering Advances
- ICSNC 2025, The Twentieth International Conference on Systems and Networks Communications
- CENTRIC 2025, The Eighteenth International Conference on Advances in Human-oriented and Personalized Mechanisms, Technologies, and Services
- VALID 2025, The Seventeenth International Conference on Advances in System Testing and Validation Lifecycle
- SIMUL 2025, The Seventeenth International Conference on Advances in System Simulation
- SOTICS 2025, The Fifteenth International Conference on Social Media Technologies, Communication, and Informatics
- INNOV 2025, The Fourteenth International Conference on Communications, Computation, Networks and Technologies
- AISyS 2025, The Second International Conference on AI-based Systems and Services
SocSys 2025 Congress October 26, 2025 to October 30, 2025 - Barcelona, Spain
NetWare 2025 Congress October 26, 2025 to October 30, 2025 - Barcelona, Spain
- SENSORCOMM 2025, The Nineteenth International Conference on Sensor Technologies and Applications
- SENSORDEVICES 2025, The Sixteenth International Conference on Sensor Device Technologies and Applications
- SECURWARE 2025, The Nineteenth International Conference on Emerging Security Information, Systems and Technologies
- AFIN 2025, The Seventeenth International Conference on Advances in Future Internet
- CENICS 2025, The Eighteenth International Conference on Advances in Circuits, Electronics and Micro-electronics
- ICQNM 2025, The Nineteenth International Conference on Quantum, Nano/Bio, and Micro Technologies
- FASSI 2025, The Eleventh International Conference on Fundamentals and Advances in Software Systems Integration
- GREEN 2025, The Tenth International Conference on Green Communications, Computing and Technologies
- HEALTHINFO 2025, The Tenth International Conference on Informatics and Assistive Technologies for Health-Care, Medical Support and Wellbeing
TechWorld 2025 Congress October 26, 2025 to October 30, 2025 - Barcelona, Spain
|
|
|
|
|
ThinkMind // CLOUD COMPUTING 2010, The First International Conference on Cloud Computing, GRIDs, and Virtualization // View article cloud_computing_2010_3_20_50031
The Limitation of MapReduce: A Probing Case and a Lightweight Solution
 Authors: Zhiqiang Ma Lin Gu Keywords: Distributed computing; Parallel architectures Abstract: MapReduce is arguably the most successful parallelization framework especially for processing large data sets in datacenters comprising commodity computers. However, difficulties are observed in porting sophisticated applications to MapReduce, albeit the existence of numerous parallelization opportunities. Intrinsically, the MapReduce design allows a program to scale up to handle extremely large data sets, but constrains a program's ability to process smaller data items and exploit variable-degrees of parallelization opportunities which are likely to be the common case in general application. In this paper, we analyze the limitations of MapReduce and present the design and implementation of a new lightweight parallelization framework, MRlite. MRlite can efficiently process moderatesize data with dependences among numerous computational steps. In the mean time, the parallelization on each step emulates the MapReduce model. Hence, the MRlite framework can also scale up for large data sets if massive parallelism with minimal dependence exists. MRlite can significantly improve the flexibility and parallel execution performance for a number of typical programs. Our evaluation shows that MRlite is one order of magnitude faster than Hadoop on problems that MapReduce has difficulty in handling. Pages: 68 to 73 Copyright: Copyright (c) IARIA, 2010 Publication date: November 21, 2010 Published in: conference ISSN: 2308-4294 ISBN: 978-1-61208-106-9 Location: Lisbon, Portugal Dates: from November 21, 2010 to November 26, 2010
|
|
SERVICES CONTACT
2010 - 2022 © ThinkMind. All rights reserved.
Read Terms of Service and Privacy Policy. |
|
|
 |